316 lines
13 KiB
Plaintext
316 lines
13 KiB
Plaintext
---
|
|
title: "Introduction to the viridis color maps"
|
|
author:
|
|
- "Bob Rudis, Noam Ross and Simon Garnier"
|
|
date: "`r Sys.Date()`"
|
|
output:
|
|
rmarkdown::html_vignette:
|
|
toc: true
|
|
toc_depth: 1
|
|
vignette: >
|
|
%\VignetteIndexEntry{Introduction to the viridis color maps}
|
|
%\VignetteEngine{knitr::rmarkdown}
|
|
%\VignetteEncoding{UTF-8}
|
|
---
|
|
|
|
<style>
|
|
img {
|
|
max-width: 100%;
|
|
max-height: 100%;
|
|
}
|
|
</style>
|
|
|
|
# tl;dr
|
|
|
|
Use the color scales in this package to make plots that are pretty,
|
|
better represent your data, easier to read by those with colorblindness, and
|
|
print well in gray scale.
|
|
|
|
Install **viridis** like any R package:
|
|
|
|
```
|
|
install.packages("viridis")
|
|
library(viridis)
|
|
```
|
|
|
|
For base plots, use the `viridis()` function to generate a palette:
|
|
|
|
```{r setup, include=FALSE}
|
|
library(viridis)
|
|
knitr::opts_chunk$set(echo = TRUE, fig.retina=2, fig.width=7, fig.height=5)
|
|
```
|
|
|
|
```{r tldr_base, message=FALSE}
|
|
x <- y <- seq(-8*pi, 8*pi, len = 40)
|
|
r <- sqrt(outer(x^2, y^2, "+"))
|
|
filled.contour(cos(r^2)*exp(-r/(2*pi)),
|
|
axes=FALSE,
|
|
color.palette=viridis,
|
|
asp=1)
|
|
```
|
|
|
|
For ggplot, use `scale_color_viridis()` and `scale_fill_viridis()`:
|
|
|
|
```{r, tldr_ggplot, message=FALSE}
|
|
library(ggplot2)
|
|
ggplot(data.frame(x = rnorm(10000), y = rnorm(10000)), aes(x = x, y = y)) +
|
|
geom_hex() + coord_fixed() +
|
|
scale_fill_viridis() + theme_bw()
|
|
```
|
|
|
|
---
|
|
|
|
# Introduction
|
|
|
|
[`viridis`](https://cran.r-project.org/package=viridis), and its companion
|
|
package [`viridisLite`](https://cran.r-project.org/package=viridisLite)
|
|
provide a series of color maps that are designed to improve graph readability
|
|
for readers with common forms of color blindness and/or color vision deficiency.
|
|
The color maps are also perceptually-uniform, both in regular form and also when
|
|
converted to black-and-white for printing.
|
|
|
|
These color maps are designed to be:
|
|
|
|
- **Colorful**, spanning as wide a palette as possible so as to make differences
|
|
easy to see,
|
|
- **Perceptually uniform**, meaning that values close to each other have
|
|
similar-appearing colors and values far away from each other have more
|
|
different-appearing colors, consistently across the range of values,
|
|
- **Robust to colorblindness**, so that the above properties hold true for
|
|
people with common forms of colorblindness, as well as in grey scale printing, and
|
|
- **Pretty**, oh so pretty
|
|
|
|
`viridisLite` provides the base functions for generating the color maps in base
|
|
`R`. The package is meant to be as lightweight and dependency-free as possible
|
|
for maximum compatibility with all the `R` ecosystem. [`viridis`](https://cran.r-project.org/package=viridis)
|
|
provides additional functionalities, in particular bindings for `ggplot2`.
|
|
|
|
---
|
|
|
|
# The Color Scales
|
|
|
|
The package contains eight color scales: "viridis", the primary choice, and
|
|
five alternatives with similar properties - "magma", "plasma", "inferno",
|
|
"civids", "mako", and "rocket" -, and a rainbow color map - "turbo".
|
|
|
|
The color maps `viridis`, `magma`, `inferno`, and `plasma` were created by
|
|
Stéfan van der Walt ([@stefanv](https://github.com/stefanv)) and Nathaniel Smith ([@njsmith](https://github.com/njsmith)). If you want to know more about the
|
|
science behind the creation of these color maps, you can watch this
|
|
[presentation of `viridis`](https://youtu.be/xAoljeRJ3lU) by their authors at
|
|
SciPy 2015.
|
|
|
|
The color map `cividis` is a corrected version of 'viridis', developed by
|
|
Jamie R. Nuñez, Christopher R. Anderton, and Ryan S. Renslow, and originally
|
|
ported to `R` by Marco Sciaini ([@msciain](https://github.com/marcosci)). More
|
|
info about `cividis` can be found in
|
|
[this paper](https://journals.plos.org/plosone/article?id=10.1371/journal.pone.0199239).
|
|
|
|
The color maps `mako` and `rocket` were originally created for the `Seaborn`
|
|
statistical data visualization package for Python. More info about `mako` and
|
|
`rocket` can be found on the
|
|
[`Seaborn` website](https://seaborn.pydata.org/tutorial/color_palettes.html).
|
|
|
|
The color map `turbo` was developed by Anton Mikhailov to address the
|
|
shortcomings of the Jet rainbow color map such as false detail, banding and
|
|
color blindness ambiguity. More infor about `turbo` can be found
|
|
[here](https://ai.googleblog.com/2019/08/turbo-improved-rainbow-colormap-for.html).
|
|
|
|
```{r for_repeat, include=FALSE}
|
|
n_col <- 128
|
|
|
|
img <- function(obj, nam) {
|
|
image(1:length(obj), 1, as.matrix(1:length(obj)), col=obj,
|
|
main = nam, ylab = "", xaxt = "n", yaxt = "n", bty = "n")
|
|
}
|
|
```
|
|
|
|
```{r begin, message=FALSE, include=FALSE}
|
|
library(viridis)
|
|
library(scales)
|
|
library(colorspace)
|
|
library(dichromat)
|
|
```
|
|
|
|
```{r show_scales, echo=FALSE, fig.height=3.575}
|
|
par(mfrow=c(8, 1), mar=rep(1, 4))
|
|
img(rev(viridis(n_col)), "viridis")
|
|
img(rev(magma(n_col)), "magma")
|
|
img(rev(plasma(n_col)), "plasma")
|
|
img(rev(inferno(n_col)), "inferno")
|
|
img(rev(cividis(n_col)), "cividis")
|
|
img(rev(mako(n_col)), "mako")
|
|
img(rev(rocket(n_col)), "rocket")
|
|
img(rev(turbo(n_col)), "turbo")
|
|
```
|
|
|
|
---
|
|
|
|
# Comparison
|
|
|
|
Let's compare the viridis and magma scales against these other commonly used
|
|
sequential color palettes in R:
|
|
|
|
- Base R palettes: `rainbow.colors`, `heat.colors`, `cm.colors`
|
|
- The default **ggplot2** palette
|
|
- Sequential [colorbrewer](https://colorbrewer2.org/) palettes, both default
|
|
blues and the more viridis-like yellow-green-blue
|
|
|
|
```{r 01_normal, echo=FALSE}
|
|
par(mfrow=c(7, 1), mar=rep(1, 4))
|
|
img(rev(rainbow(n_col)), "rainbow")
|
|
img(rev(heat.colors(n_col)), "heat")
|
|
img(rev(seq_gradient_pal(low = "#132B43", high = "#56B1F7", space = "Lab")(seq(0, 1, length=n_col))), "ggplot default")
|
|
img(gradient_n_pal(brewer_pal(type="seq")(9))(seq(0, 1, length=n_col)), "brewer blues")
|
|
img(gradient_n_pal(brewer_pal(type="seq", palette = "YlGnBu")(9))(seq(0, 1, length=n_col)), "brewer yellow-green-blue")
|
|
img(rev(viridis(n_col)), "viridis")
|
|
img(rev(magma(n_col)), "magma")
|
|
```
|
|
|
|
It is immediately clear that the "rainbow" palette is not perceptually uniform;
|
|
there are several "kinks" where the apparent color changes quickly over a short
|
|
range of values. This is also true, though less so, for the "heat" colors.
|
|
The other scales are more perceptually uniform, but "viridis" stands out for its
|
|
large *perceptual range*. It makes as much use of the available color space as
|
|
possible while maintaining uniformity.
|
|
|
|
Now, let's compare these as they might appear under various forms of colorblindness,
|
|
which can be simulated using the **[dichromat](https://cran.r-project.org/package=dichromat)**
|
|
package:
|
|
|
|
### Green-Blind (Deuteranopia)
|
|
|
|
```{r 02_deutan, echo=FALSE}
|
|
par(mfrow=c(7, 1), mar=rep(1, 4))
|
|
img(dichromat(rev(rainbow(n_col)), "deutan"), "rainbow")
|
|
img(dichromat(rev(heat.colors(n_col)), "deutan"), "heat")
|
|
img(dichromat(rev(seq_gradient_pal(low = "#132B43", high = "#56B1F7", space = "Lab")(seq(0, 1, length=n_col))), "deutan"), "ggplot default")
|
|
img(dichromat(gradient_n_pal(brewer_pal(type="seq")(9))(seq(0, 1, length=n_col)), "deutan"), "brewer blues")
|
|
img(dichromat(gradient_n_pal(brewer_pal(type="seq", palette = "YlGnBu")(9))(seq(0, 1, length=n_col)), "deutan"), "brewer yellow-green-blue")
|
|
img(dichromat(rev(viridis(n_col)), "deutan"), "viridis")
|
|
img(dichromat(rev(magma(n_col)), "deutan"), "magma")
|
|
```
|
|
|
|
### Red-Blind (Protanopia)
|
|
|
|
```{r 03_protan, echo=FALSE}
|
|
par(mfrow=c(7, 1), mar=rep(1, 4))
|
|
img(dichromat(rev(rainbow(n_col)), "protan"), "rainbow")
|
|
img(dichromat(rev(heat.colors(n_col)), "protan"), "heat")
|
|
img(dichromat(rev(seq_gradient_pal(low = "#132B43", high = "#56B1F7", space = "Lab")(seq(0, 1, length=n_col))), "protan"), "ggplot default")
|
|
img(dichromat(gradient_n_pal(brewer_pal(type="seq")(9))(seq(0, 1, length=n_col)), "protan"), "brewer blues")
|
|
img(dichromat(gradient_n_pal(brewer_pal(type="seq", palette = "YlGnBu")(9))(seq(0, 1, length=n_col)), "protan"), "brewer yellow-green-blue")
|
|
img(dichromat(rev(viridis(n_col)), "protan"), "viridis")
|
|
img(dichromat(rev(magma(n_col)), "protan"), "magma")
|
|
```
|
|
|
|
### Blue-Blind (Tritanopia)
|
|
|
|
```{r 04_tritan, echo=FALSE}
|
|
par(mfrow=c(7, 1), mar=rep(1, 4))
|
|
img(dichromat(rev(rainbow(n_col)), "tritan"), "rainbow")
|
|
img(dichromat(rev(heat.colors(n_col)), "tritan"), "heat")
|
|
img(dichromat(rev(seq_gradient_pal(low = "#132B43", high = "#56B1F7", space = "Lab")(seq(0, 1, length=n_col))), "tritan"), "ggplot default")
|
|
img(dichromat(gradient_n_pal(brewer_pal(type="seq")(9))(seq(0, 1, length=n_col)), "tritan"), "brewer blues")
|
|
img(dichromat(gradient_n_pal(brewer_pal(type="seq", palette = "YlGnBu")(9))(seq(0, 1, length=n_col)), "tritan"), "brewer yellow-green-blue")
|
|
img(dichromat(rev(viridis(n_col)), "tritan"), "viridis")
|
|
img(dichromat(rev(magma(n_col)), "tritan"), "magma")
|
|
```
|
|
|
|
### Desaturated
|
|
|
|
```{r 05_desatureated, echo=FALSE}
|
|
par(mfrow=c(7, 1), mar=rep(1, 4))
|
|
img(desaturate(rev(rainbow(n_col))), "rainbow")
|
|
img(desaturate(rev(heat.colors(n_col))), "heat")
|
|
img(desaturate(rev(seq_gradient_pal(low = "#132B43", high = "#56B1F7", space = "Lab")(seq(0, 1, length=n_col)))), "ggplot default")
|
|
img(desaturate(gradient_n_pal(brewer_pal(type="seq")(9))(seq(0, 1, length=n_col))), "brewer blues")
|
|
img(desaturate(gradient_n_pal(brewer_pal(type="seq", palette = "YlGnBu")(9))(seq(0, 1, length=n_col))), "brewer yellow-green-blue")
|
|
img(desaturate(rev(viridis(n_col))), "viridis")
|
|
img(desaturate(rev(magma(n_col))), "magma")
|
|
```
|
|
|
|
We can see that in these cases, "rainbow" is quite problematic - it is not
|
|
perceptually consistent across its range. "Heat" washes
|
|
out at bright colors, as do the brewer scales to a lesser extent. The ggplot scale
|
|
does not wash out, but it has a low perceptual range - there's not much contrast
|
|
between low and high values. The "viridis" and "magma" scales do better - they cover a wide perceptual range in brightness in brightness and blue-yellow, and do not rely as much on red-green contrast. They do less well
|
|
under tritanopia (blue-blindness), but this is an extrememly rare form of colorblindness.
|
|
|
|
---
|
|
|
|
# Usage
|
|
|
|
The `viridis()` function produces the `viridis` color scale. You can choose
|
|
the other color scale options using the `option` parameter or the convenience
|
|
functions `magma()`, `plasma()`, `inferno()`, `cividis()`, `mako()`, `rocket`()`,
|
|
and `turbo()`.
|
|
|
|
Here the `inferno()` scale is used for a raster of U.S. max temperature:
|
|
|
|
```{r tempmap, message=FALSE}
|
|
library(terra)
|
|
library(httr)
|
|
par(mfrow=c(1,1), mar=rep(0.5, 4))
|
|
temp_raster <- "http://ftp.cpc.ncep.noaa.gov/GIS/GRADS_GIS/GeoTIFF/TEMP/us_tmax/us.tmax_nohads_ll_20150219_float.tif"
|
|
try(GET(temp_raster,
|
|
write_disk("us.tmax_nohads_ll_20150219_float.tif")), silent=TRUE)
|
|
us <- rast("us.tmax_nohads_ll_20150219_float.tif")
|
|
us <- project(us, y="+proj=aea +lat_1=29.5 +lat_2=45.5 +lat_0=37.5 +lon_0=-96 +x_0=0 +y_0=0 +ellps=GRS80 +datum=NAD83 +units=m +no_defs")
|
|
image(us, col=inferno(256), asp=1, axes=FALSE, xaxs="i", xaxt='n', yaxt='n', ann=FALSE)
|
|
```
|
|
|
|
The package also contains color scale functions for **ggplot**
|
|
plots: `scale_color_viridis()` and `scale_fill_viridis()`. As with `viridis()`,
|
|
you can use the other scales with the `option` argument in the `ggplot` scales.
|
|
Here the "magma" scale is used for a cloropleth map of U.S. unemployment:
|
|
|
|
```{r, ggplot2}
|
|
library(maps)
|
|
library(mapproj)
|
|
|
|
data(unemp, package = "viridis")
|
|
|
|
county_df <- map_data("county", projection = "albers", parameters = c(39, 45))
|
|
names(county_df) <- c("long", "lat", "group", "order", "state_name", "county")
|
|
county_df$state <- state.abb[match(county_df$state_name, tolower(state.name))]
|
|
county_df$state_name <- NULL
|
|
|
|
state_df <- map_data("state", projection = "albers", parameters = c(39, 45))
|
|
|
|
choropleth <- merge(county_df, unemp, by = c("state", "county"))
|
|
choropleth <- choropleth[order(choropleth$order), ]
|
|
|
|
ggplot(choropleth, aes(long, lat, group = group)) +
|
|
geom_polygon(aes(fill = rate), colour = alpha("white", 1 / 2), linewidth = 0.2) +
|
|
geom_polygon(data = state_df, colour = "white", fill = NA) +
|
|
coord_fixed() +
|
|
theme_minimal() +
|
|
ggtitle("US unemployment rate by county") +
|
|
theme(axis.line = element_blank(), axis.text = element_blank(),
|
|
axis.ticks = element_blank(), axis.title = element_blank()) +
|
|
scale_fill_viridis(option="magma")
|
|
```
|
|
|
|
The ggplot functions also can be used for discrete scales with the argument
|
|
`discrete=TRUE`.
|
|
|
|
```{r discrete}
|
|
p <- ggplot(mtcars, aes(wt, mpg))
|
|
p + geom_point(size=4, aes(colour = factor(cyl))) +
|
|
scale_color_viridis(discrete=TRUE) +
|
|
theme_bw()
|
|
```
|
|
|
|
# Gallery
|
|
|
|
Here are some examples of viridis being used in the wild:
|
|
|
|
James Curley uses **viridis** for matrix plots ([Code](https://gist.github.com/jalapic/9a1c069aa8cee4089c1e)):
|
|
|
|
[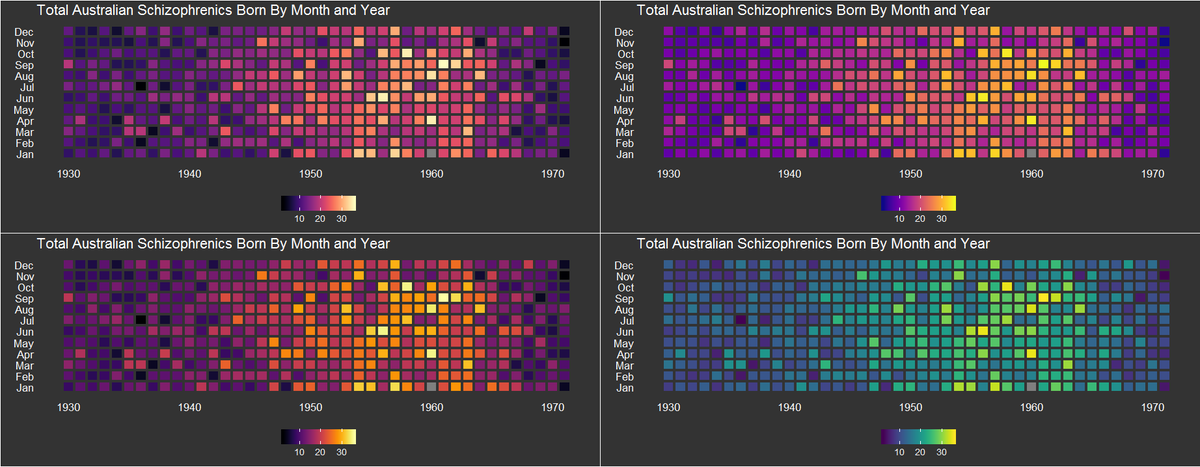](http://pbs.twimg.com/media/CQWw9EgWsAAoUi0.png)
|
|
|
|
Christopher Moore created these contour plots of potential in a dynamic
|
|
plankton-consumer model:
|
|
|
|
[](http://pbs.twimg.com/media/CQWTy7wWcAAa-gu.jpg)
|